Will ChatGPT make us better, happier teachers?
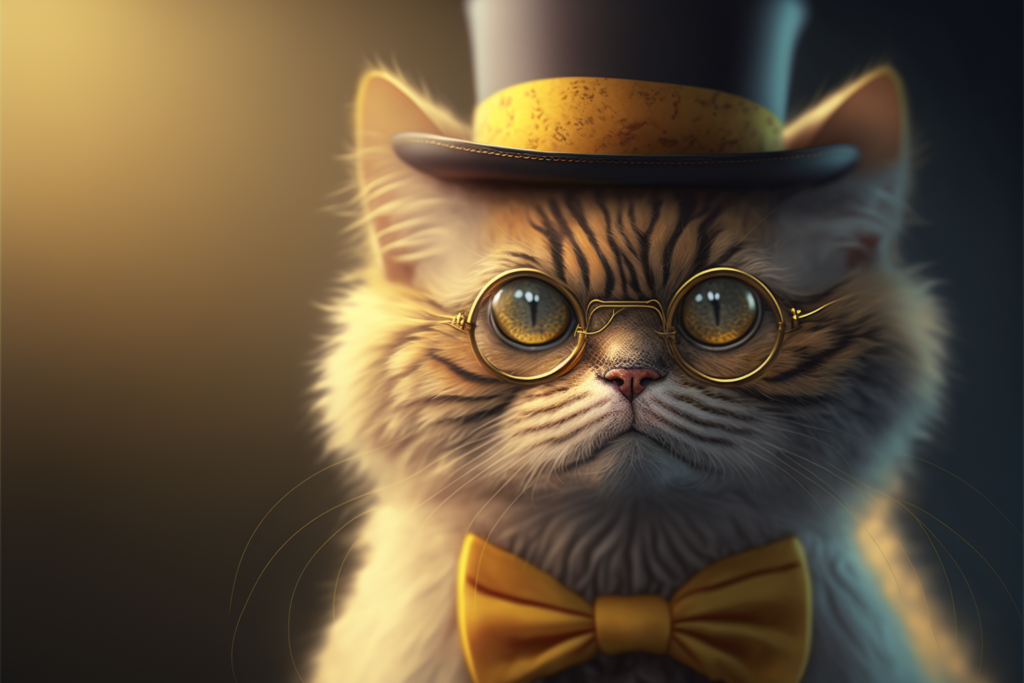
When I was six years old, my most prized possession was a “Smarty Bear.” Unlike the better-known Teddy Ruxpin, which read a taped script, Smarty Bear was an animatronic teddy bear that would interact and respond to my questions. Over time, I grew disenchanted with his eight-ball-like answers and asked Santa to develop an even better toy that did not yet exist: a walking, talking Garfield that was actually alive. Although this request was denied (1980s elves had their limits!), I’ve been holding out hope for artificial intelligence ever since.
No one who has talked with me in the last month will be surprised by this story.1 Like so many, I have been completely captivated by ChatGPT and its image-generating cousins. My interests in artificial intelligence are wide-ranging (did you know we’ve taught neurons in a petri dish to play Pong??). But I have, for obvious reasons, been particularly interested in conversations among educators about what ChatGPT means for the future of teaching and learning. And if you’ve been following these conversations, as well, you know there is a lot being said.
Yes, ChatGPT makes major and sometimes comical mistakes. No, it is not always possible to distinguish its output from that of a human. Yes, students are probably going to use ChatGPT to assist with their academic work. No, it’s not clear this will always be “cheating.” Yes, students can use ChatGPT to develop their knowledge and critical thinking skills. No, this does not mean ChatGPT is always beneficial for learning. Yes, we can make our assessments ChatGPT-proof (for now). No, we cannot make such changes without important pedagogical tradeoffs. Yes, we are probably overly focused on cheating. No, it will not be enough to simply “trust students” and design assignments that are “worth doing.”
These quick takes align with my considered positions, but none of them will be the focus of this particular post. Any serious accounting for them would require thousands of words, and it is not clear I can add much to what has already been said by those far smarter than me. So, Instead, I want to address a set of questions that has been relatively under-discussed.2 More specifically, I want to turn our attention from what ChatGPT can do for students to thinking about what it might do for their teachers.
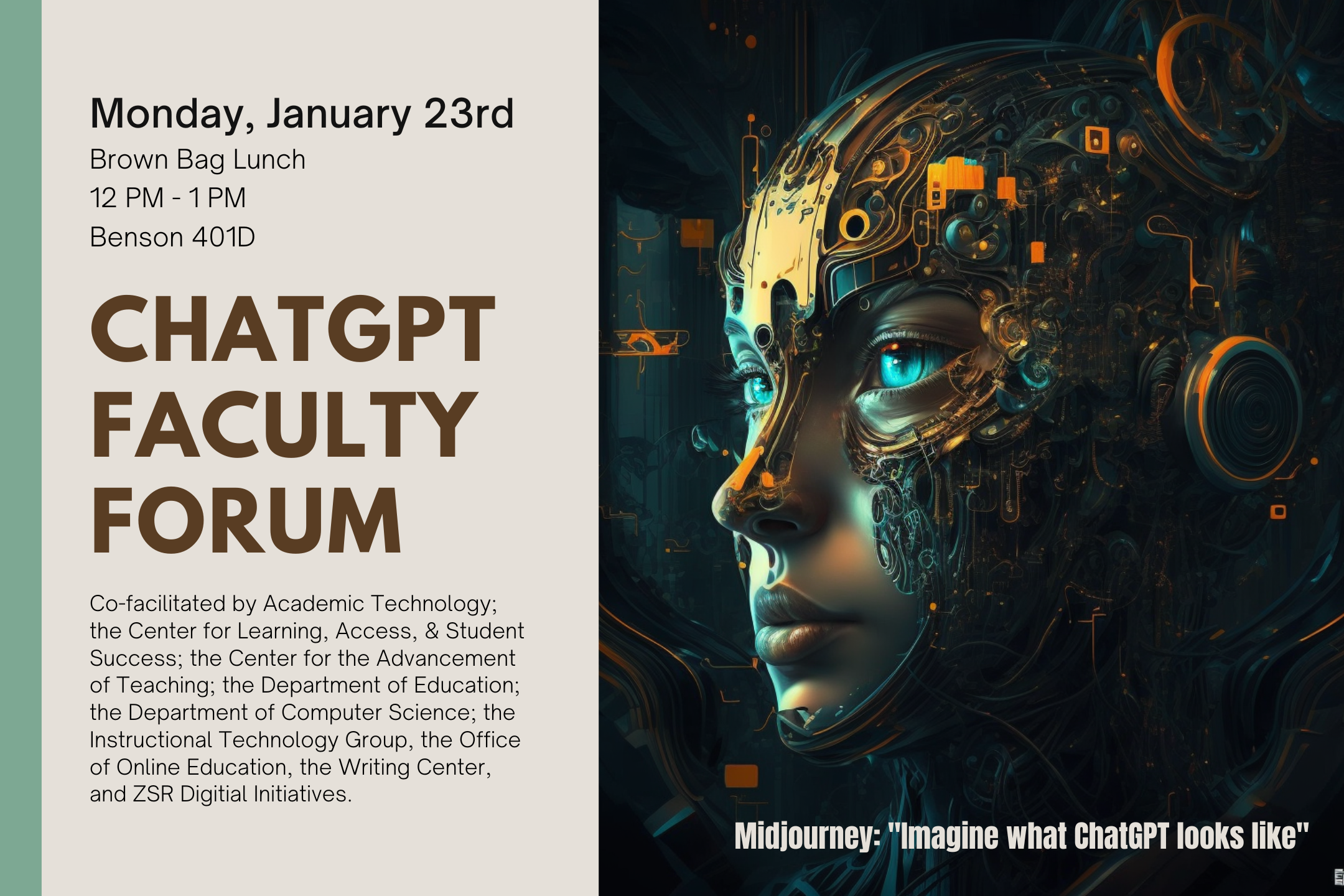
If you still have questions about student use, we encourage you to grab your lunch and join us for a ChatGPT Faculty Forum on Monday, January 23rd. We’ll share resources, give you an opportunity to experiment with the tool, and discuss its implications for student learning and academic integrity.
I am old enough to remember my first email and know exactly where I was when I used my first smartphone app. I will never forget my first encounter with ChatGPT, either. Fittingly for me, it came in the form of a syllabus. I was writing emails in my office when I got the following message from my colleague Kyle Denlinger, in Slack:
I’ve been playing around with ChatGPT, the AI chatbot that everyone’s been talking about. Look what it made for me in the span of 5 seconds, based on my simple prompt. It was nice working with you all.
Curious, I opened the attachment and read with increasingly wide eyes:
I’m sure I would have discovered ChatGPTs course design skills eventually. Like Kyle and the rest of the working world, I’d want to know whether it could do my job. But thanks to Kyle’s prompting, I’ve had something of a head start. And I’ve spent the last month experimenting with ChatGPT’s ability to assist with our teaching.
Some of this experimentation has involved exploring its ability to directly support our students and their learning. As many have already noted, it can be useful as a tool for explaining or re-explaining complicated material in ways that make sense to the individual student (if you haven’t already done this, ask it to explain your research to a third grader; it’s delightful!). And while the feedback it provides on student work may not be good enough to replace that of an expert, it can be fruitful for immediate and ongoing self-assessment (see this example, which hilariously suggests itself!).
But I am most excited about ChatGPT’s ability to support the essential work of learning design.
In The Learner-Centered Teacher (2011), Terry Doyle advocates for active, engaged learning by arguing that “the one who does the work does the learning.” His point is that students are unlikely to learn if their instructors are the ones doing most of the work in a particular class period. It’s an important point, but slightly oversimplified. As Steven Chu has recently argued, student engagement is not synonymous with student learning. While engagement may be a necessary condition for learning, it is not sufficient. The engagement must be carefully crafted by the instructor to promote learning. But this means the work of the instructor is just as important as the work of the student. And as anyone who has designed a course will tell you, that work is no joke.
So, given the importance of course design to good teaching, and how difficult that work actually is, how might ChatGPT lend a hand? By helping us brainstorm disciplinary-relevant ideas at each step of the traditional design process (outcomes, assessments, and learning activities), and by helping us when we’re stuck trying to figure out why our design is just not working as we’d hoped.
Crafting Student Learning Outcomes
In a scathing Chronicle essay from early January, Gayle Greene expressed the views of many faculty when she critiqued the “terrible tedium of learning outcomes.” Some of the discontent is tied to a mistaken understanding of the purpose of the task. If you believe we only craft learning outcomes to satisfy external accreditors, it makes sense to be grumpy. But even those of us who believe this task is essential to our work may still find it tedious. And that’s because translating our goals into concrete learning outcomes is difficult, time-consuming work.
Part of the issue is that, as experts, our aspirations for students are usually very specific but also largely intuitive. We have a hard time articulating them but an easy time explaining why various formulations are not quite right. What we need most are very specific examples, but the poor schmucks at the teaching center usually only have examples from unrelated disciplines or courses in our discipline we’re not actually teaching. It can be hard to see past the substance of those examples to their linguistic structure, making it difficult to translate our intuitions into anything similar.
Yet translating is just the kind of thing a large language model like ChatGPT can do exceptionally well. And within minutes of seeing the syllabus ChatGPT produced for Kyle, I was asking it to craft learning outcomes for various kinds of obscure courses. I even went to our university catalog and began asking it to translate the vague, 150-word course descriptions into operationalized outcomes (see this, this, and this). The results were not always great. But I’ve read a lot of student learning outcomes written by actual humans, and I’m here to tell you: ChatGPT has us beat.
Some might worry that using ChatGPT in this way will reinforce the idea that this entire enterprise is meaningless box-checking. And I’m guessing Gayle Green would argue that ChatGPT’s ability to do this is evidence that formulating operationalized learning outcomes is not a serious intellectual task. But in this case, the value of the task does not rest in its complexity (nor does ChatGPT’s ability to do it well mean it is simple). As with most translating, the task is valuable because it is necessary to achieve other valuable goals.
Assignments & Activities
The primary value of carefully formulated learning outcomes is that they make all the other work of course design possible. Without a clear sense of what we want students to learn, it is harder to design non-arbitrary assessments, and our learning activities are less likely to be effective. To use an analogy my colleague Jerod Quinn likes to use, it’s like packing for a vacation without knowing where you’re going. That parka might be useful. But it might also be a total waste of space.
In other words, learning outcomes provide something of a roadmap for the rest of the course design process. And do you know what does well with roadmaps? Computers.
Sure enough, when prompted with specific outcomes, ChatGPT is able to brainstorm assignments that will assess those outcomes (see this, this, and this) and activities that will help our students achieve them (see this, this, and this). Some assessments and activities come with grading criteria, and if you ask for a “lesson plan,” you get activities, assessments, and a schedule rolled into one (see this and this).
As these are more complex tasks less tied to language, the results are somewhat less impressive than they were for learning outcomes. So far as I can tell, the assignments and strategies it suggests are relatively basic. But of the examples I understand (differential equations not included!), I have yet to read one that I thought would be ineffective or misaligned with the outcome–something that cannot be said of many human-generated assignments and activities.
Solving Teaching Challenges
As a large language model trained on a huge swath of information from the pre-2021 internet, ChatGPT “knows” quite a bit. It’s also relatively good at synthesizing all that information, and remarkably good at predicting which synthesis is the most appropriate response to specific prompts. So, unlike Google, which answers our questions by giving us a list of potential sources, ChatGPT just answers them (you can download an extension in Chrome that allows you to compare their responses). If it were just copying from a website, Google’s list would be vastly superior. But it’s not copying from a single source. It’s synthesizing all the relevant sources in its training material.
All of which is to say ChatGPT is at its best when you ask it a relatively straightforward question about which many people have already written. And as much as I don’t want to admit this, a good part of what I do in consultations with faculty is answer relatively straightforward questions about which many people have already written. So I knew, even before I experimented, that ChatGPT was going to be an excellent source of information about evidence-informed teaching strategies.
Teaching is challenging for many reasons, but one is that even a well-designed course can sometimes fall flat. We have a clear idea about how we want the class to proceed and what we think the students will learn. But the class gets stuck, and the students aren’t learning. While some challenges can be idiosyncratic, most are relatively common and widely researched. But faculty don’t have the time to do a literature review when they’re scrambling to prepare for their next class period.
This has, historically, been one major benefit of an active teaching center like the CAT. My colleagues and I have read the literature for you and are just a quick trip or phone call away. Now, it seems, we may have a new colleague.
While ChatGPT cannot do everything we do, and I would want to ask for more context before I share strategies, it does seem especially good at capturing and communicating a wide range of evidence-informed responses to common teaching problems. It can, among other things, help you formulate questions to encourage discussion; incentivize students to do the assigned reading before class; encourage more than two students to participate; and help students manage group projects to avoid the free-rider problem.
OK. But.
This post has been relatively enthusiastic about ChatGPT, but there are legitimate concerns about the approach I am advocating. We might reasonably worry that using a machine to support course design could lead us astray, diminish the value of pedagogical creativity and expertise, or hasten a dystopian future where human teachers are replaced by machines.
As impressive as ChatGPT may be, it is important for all of us to understand that large language models are not actually thinking. As a result, they are not–and may never be–a substitute for human expertise. In fact, the approach I am advocating depends on that expertise. These models can generate outputs, but only an expert can assess, edit, integrate, and refine those outputs to produce an ideal result.
For all of these reasons, we should proceed with caution. But used wisely, ChatGPT may actually make our teaching more rather than less humane. By using AI to streamline our analytic tasks, we can devote more time to fostering deeper connections with our students – connections that not only benefit them, but also serve as a much-needed source of rejuvenation for educators who have been stretched thin by years of teaching during a pandemic. In this sense, ChatGPT can be seen as a gift – a tool that can help us reconnect with our students and reignite our passion for teaching.3
- Nor will anyone be surprised that a cat plays a leading role.
- K-12 teachers have been talking about this for quite some time. As usual, we have much to learn from them.
- This paragraph, and only this paragraph, was written with assistance from ChatGPT and Ezra Klein. Both were unaware.
Subscribe
Receive CAT blog posts in your inbox.