Syllabus Week in the Age of AI: Part I
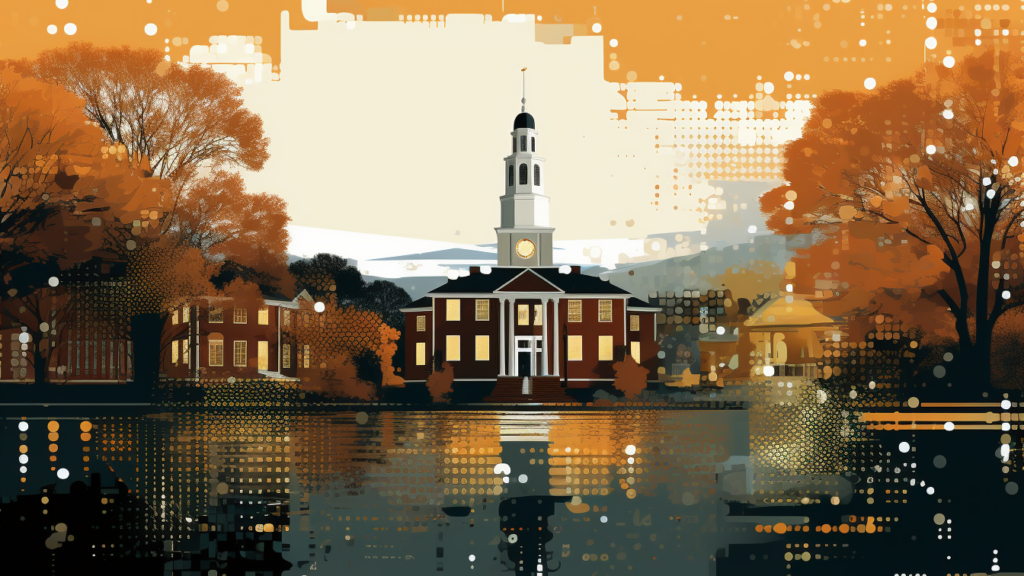
For teaching centers, the weeks before the start of the fall semester are the most wonderful time of the year. We welcome new faculty into our community of teacher-scholars, and faculty of all ranks begin to reach out as they design their syllabi and reflect on changes they would like to make. This year, almost everyone is considering how they want to approach artificial intelligence in their classrooms. Although ChatGPT was released in November, it took some time for the news to spread, and many of us took a wait-and-see approach in the spring. We’re now hoping to develop a more intentional approach. Yet, as is often the case in the life of a teacher, there is too much to read and not enough time.
This post is the first of a four-part series that aims to ease that burden by summarizing and curating the relevant literature. Given the need for just-in-time guidance, I’ve structured each post as a series of FAQs with the most time-sensitive questions at the top. Today’s post will address AI policy, syllabus statements, and strategies for preserving academic integrity. In later posts, I’ll share strategies for using AI to support student learning, discuss ways AI might support our work as teachers, and recommend readings, podcasts, and videos for those who want to learn more about the nature of artificial intelligence and its broader implications for society.
Although I am introducing these FAQs in blog posts, they will also live together on our new AI Resources page. We will continue to update those resources throughout the year, so we encourage you to bookmark that page for future reference.
As always, the CAT is available for consultations if you have any questions or would like a conversation partner as you think through your approach!
Just-In-Time Guidance
First, you should know that you are not alone. Given the pace of these developments, very few of us have a complete handle on what these tools can do and why they matter. As one of my favorite comics points out, even the experts who developed these models aren’t quite sure what is happening under the hood. We’re all feeling a bit like deer in headlights, so give yourself some grace.
Second, if we learned anything from our experience of emergency remote teaching in 2020, it is that we are better together. When we joined formal and informal learning communities to ask questions, share ideas, and reflect on what did and did not work, the load felt lighter. So now, as ever, I recommend joining your colleagues in conversations about how they are approaching AI in their classrooms.
This can happen within your departments, but the CAT will continue to facilitate opportunities for interdisciplinary collaboration. In addition to ai consultations and workshops, we’ve created a WFU AI and Education Google Group to allow faculty to share news, questions, and useful examples. We will continue to share resources we have found via our newsletter and blog, and hope to develop larger-scale programs later this year.
If you find yourself curious about what is happening at other institutions, you can also join the “AI in Higher Education” Google Group developed by our colleagues in the POD Network or the “Higher Ed Discussions of AI Writing” Facebook Group, developed by Laura Dumin, Professor of Technical Writing and AI Coordinator for the University of Central Oklahoma. If you enjoy Substack newsletters, I can also recommend signing up for updates from Ethan Mollick at Penn and Marc Watkins at the University of Mississippi.
The University has not yet established an institutional policy governing AI use on campus. Our leaders have chosen (wisely, I think) to hold off on such a policy until we have a better understanding of these tools and the various ways our faculty and students are using them. Instead, schools, departments, course coordinators, and individual instructors have been encouraged to develop approaches aligned with their pedagogical goals.
As a result, most instructors will be free to take whatever approach they prefer, including no approach at all. Yet an environment that welcomes various–and sometimes conflicting–policies is also an environment that benefits from transparency. Without a “default” approach to AI on campus, communication between faculty and students will be even more essential than it already is. For this reason, my number one recommendation for faculty this fall is to be as transparent as possible about your specific approach to AI and how it may differ from other approaches on campus.
If you’re genuinely unsure of what your approach should be, share this with your students. You might also consider it a pedagogical opportunity. We know policies are more likely to be followed when they are co-constructed with our students, so you could spend the first few weeks working together to develop your approach.
Before you can draft your syllabus statement, you must first decide when AI use is and is not acceptable in your course. In an ideal world, you would become an AI expert before making those decisions. In the real world, it is probably enough to ask yourself whether the various uses outlined in this worksheet would be problematic in your course.
From a teaching and learning perspective, the most important question you should be asking is: “Does this particular use of AI support or undermine the knowledge, skills, and dispositions I aim to develop in my course?” Without clearly defined outcomes, this can be a tricky question to answer. But once you’ve clarified your goals, you will immediately understand why we cannot have a one-size-fits-all AI policy. If I hope to develop students’ ability to edit sentences for clarity, my students will need to practice editing sentences independently. But if I want them to develop their ability to explain this process, they could benefit from using AI to quiz themselves and check their understanding. And if I am to develop both skills, I will need to craft a nuanced, conditional policy.
For further practical guidance on drafting statements, see:
- Gannon, Kevin. “Should You Add an AI Policy to Your Syllabus?” Chronicle of Higher Education. July 31, 2023.
- “Considerations on Wording When Creating Advice or Policy on AI Use.” National Centre for AI (blog). February 14, 2023.
- Watkins, Ryan. “Update Your Course Syllabus for ChatGPT.” Medium (blog). December 19, 2022.
Yes, many!
Lance Eaton, the Director of Digital Pedagogy for College Unbound, curates the definitive archive of sample AI policies. The CAT has also curated a Wake-Forest-specific archive that brings together examples from the School of Law, School of Business, and numerous departments within the College. We hope this list will continue to grow throughout the week, so please send us your policies when you’ve finished them!
I also recommend the suggestions drafted or curated by the following Centers for Teaching & Learning:
Cheating has always existed, often at depressingly high levels.1 And for almost as long, we have sought to limit the harm it can cause through various forms of punishment (or, as we like to say now, “accountability”). In the case of cheating, punishment serves at least two functions. It stops the cheater from doing wrong (receiving an unfair advantage over fellow students) and deters other students from attempting something similar. But in both cases, detection is an essential piece of the puzzle. If we don’t know students are cheating, we can neither stop the wrongdoing nor deter others from doing the same.
There has typically been an inverse relationship between the cost of cheating and its ability to be detected. So while it has always been possible to pay another person to write an undetectable essay for you, the cost of doing so has been prohibitive for most students.2 AI presents a unique threat to the accountability approach because it changes the slope of this relationship between cost and detectability. While it’s true students must put forth some effort (they cannot, as some students have done, submit work that begins, “As a generative AI model …”), it no longer takes much work to cheat in undetectable ways.
Given this reality, it makes sense to think of the challenge before us as one of detection. If we could find a way to detect AI-generated output like we detect plagiarized papers, students would be no more likely to use AI than they are to plagiarize, and we could return to business as usual. So we seek tips to improve our ability to spot AI-generated text or, failing that, software that will do this work for us.
Unfortunately, AI detection is both technically and ethically complex, and this complexity is only going to increase with time. But even if we decide AI detection is neither realistic nor ethical, we need not despair. And that’s because accountability is not the only way to shape student behavior. Yes, punishment can be a powerful motivator. But we also know it can also have unintended and unpredictable effects. As a result, experts on Academic Integrity have long sought to expand our toolkit beyond accountability alone. By turning our attention to these approaches, which aim to cultivate students’ positive, intrinsic motivations, it may be possible to escape the ruin of the spring semester without solving the detection problem.
- https://academicintegrity.org/resources/facts-and-statistics
- This is, of course, another example of privileged students using their privilege to extend their advantages.
Although we all want to believe we can spot AI-generated text when we encounter it, researchers have known for quite some time that humans struggle to distinguish between human- and ai-generated prose.3 It is, then, unsurprising that numerous start-ups (and OpenAI itself) were prepared to launch AI detection tools within weeks of ChatGPT’s release. And in April, Turnitin released its own secure, LMS-based tool to the faculty of over 10,000 institutions.
Since then, debates about their reliability have raged, OpenAI has quietly removed its detector from its site, Turnitin has updated its reported false-positive rates, and both Vanderbilt and The University of Pittsburgh have decided to disable Turnitin’s AI detection tools, as a result. Despite these criticisms, thousands of instructors–including many Wake Forest faculty–continue to find these tools valuable.
As with most debates, the details are more complicated than the public discourse suggests. Turnitin still maintains a 1% false positive rate for paper-level scores higher than 20%, and they are the only company able to test its tool on a 20-year archive of papers written by college students before AI came on the scene. Nevertheless, they acknowledge they will miss at least 15% of AI-generated text to maintain a false-positive rate of 1%. And this performance only applies to essays written with GPT-3. Students who can pay $20 a month for GPT-4 are far less likely to be detected.
These rates may seem encouraging if we imagine 1 false accusation for every 100 suspicious cases. Yet this false-positive rate is based on all papers submitted to Turnitin, including those we would have never investigated without the software. Assuming 25,000 papers are submitted to Turnitin each academic year, and 75% of those papers are human-generated, 188 of those human-generated papers would be inaccurately flagged as more than 20% AI-generated each year. To make this more concrete, any instructor who assigns three papers to 50 students would likely encounter 1-2 false positives each term.
Finally, it is worth remembering that AI detectors are themselves AI tools, trained in much the same way. To the extent you find AI problematic because of its propensity to”bullshit” (in Harry Frankfurt’s technical sense of that term), you must also acknowledge that these detectors are just as likely to speak confidently about things they don’t actually “know.” If you’re worried about your students trusting a machine that can hallucinate facts, remember that the same could be true of the reports you receive about student papers.
- Clark, Elizabeth, Tal August, Sofia Serrano, Nikita Haduong, Suchin Gururangan, and Noah A. Smith. 2021. “All That’s `Human’ Is Not Gold: Evaluating Human Evaluation of Generated Text.” In Proceedings of the 59th Annual Meeting of the Association for Computational Linguistics and the 11th International Joint Conference on Natural Language Processing (Volume 1: Long Papers), 7282–96. Online: Association for Computational Linguistics. https://doi.org/10.18653/v1/2021.acl-long.565; Kreps, Sarah, R. Miles McCain, and Miles Brundage. 2022. “All the News That’s Fit to Fabricate: AI-Generated Text as a Tool of Media Misinformation.” Journal of Experimental Political Science 9 (1): 104–17. https://doi.org/10.1017/XPS.2020.37.
If you’ve spent any time talking with me about the assessment of teaching, you will know that I am a big fan of compiling multiple sources of evidence when no single piece of evidence is strong. So if we want to use AI detection scores responsibly, we should always verify them with additional evidence. But what else can you use?
Some have argued that there are tell-tale signs of AI-generated text within the prose. I would be hesitant to use style as a marker, but if you discover invented sources or facts, or the passage begins “As a generative AI model, …” you can be relatively confident AI was involved. You can also introduce “verification” assignments that produce additional evidence. You have almost infinite options here, but you might consider:
- Collecting in-person writing samples to compare with out-of-class writing.
- Scheduling 1:1 conferences with students to discuss their writing process after they submit.
- Asking students to discuss their essays and writing processes with their peers.
- Giving short, in-person quizzes that ask them questions about essays they have just submitted.
- Asking students to use what they’ve learned on their out-of-class assignment to complete an in-class activity.
Although these assignments will allow you to verify the authenticity of student work, they will also be meaningful opportunities for students to practice, reflect, and improve.
You may be tempted to “AI-proof” your course by designing assignments AI can’t do (or can’t do well). Yet this approach will require you to hit a rapidly moving target as AI tools advance in unexpected ways. We once thought prompts about contemporary events were safe, but now that Bing and GPT-4 have internet access, they can handle them with ease. What is difficult today will be easy tomorrow, so you might as well assume these tools can do anything you ask.
Yet even an all-powerful AI will be useless to students if they don’t have access to it. So those of us teaching residential courses can also shift the most important activities and assessments to an in-person environment with limited access to computers and phones. We can flip our classrooms, introduce oral exams, or opt for blue-book exams instead of essays.
Yet these decisions are not without costs. Although flipped classrooms are more effective than traditional lectures, they ask a lot of the instructor the first semester the course is taught. The skills we assess in a timed writing exam are quite different from what we assess in a long-form paper written and revised over a series of weeks. And oral exams can be both time-intensive for faculty and anxiety-inducing for students.
Although punishment is a powerful extrinsic motivator, grades can be just as powerful for many of our students. There are at least two ways you can use this to your advantage.
First, you can begin the semester with an activity demonstrating the dangers of using AI to produce graded work. For this to be effective, you want the output to seem impressive. Students will be able to identify obvious hallucinations. But if you can show them they may not know enough to see the mistakes you will see, they may be less willing to rely on AI for graded work.
Second, you can structure your formative and summative assessments to motivate students to complete their take-home assignments on their own. If you expect students to demonstrate certain skills on a proctored final, and earlier take-home assignments are opportunities to practice those skills, they may be more likely to approach these assignments as genuine learning opportunities.
I will be taking the second approach in my class this fall. I know it’s not perfect (I will be giving a high-stakes, in-person exam for the first time in years, and I risk reinforcing an orientation that prioritizes grades over learning), but it gives me the freedom to assign meaningful work outside of class without submitting their work to detectors.
In an ideal world, our students would be intrinsically motivated to adhere to our guidelines, and for the right reasons. Yet they enter our classrooms with a variety of motivations, and not all of them are aligned with our goals. One might reasonably ask how much we can shape these motivations in the course of a single semester. If students don’t want to learn and care little about academic integrity, is there much we can do?
The primary reason I am optimistic about the future of AI in our classrooms is that I believe in the power of teachers. While we may not be able to win over every student, I believe most students want to learn and will do so with integrity if the conditions are right. And thanks to the fabulous work of many brilliant social scientists, we happen to know a thing or two about what those conditions look like.
For starters, we can involve them in the process of thinking through our collective approach to AI. We know that motivation increases when students feel the environment is supportive and aligned with their goals. Giving them a say in the process gives them some ownership over their environment while helping them better understand the reasons for taking a particular approach.
We also know that moral reminders can be powerful tools to motivate students to align their behavior with their values and commitments. So if we ask students to sign on to a co-constructed set of principles, and remind them of the importance of those principles before each assignment is submitted, they may be more likely to give us their best.
If you think back to the times in your life you were learning the most, what was your primary driver? Chances are it was not a desire for an A or a desire to comply with an externally imposed policy. It was, most likely, the joy of participating in activities you found personally or socially meaningful. Likewise, our courses become more meaningful when we connect our material to the interests of our students and develop relevant, authentic assignments.
Finally, it is worth noting that even the most highly motivated students, committed to learning for its own sake, can also be deeply concerned about grades. And insofar as they perceive a threat to those grades, their intrinsic motivation to learn may take a back seat. So it may not be enough to make learning meaningful in the age of AI. We may also need to reduce the power of extrinsic motivators like grades.